Meet Dr. Falah Al Amro
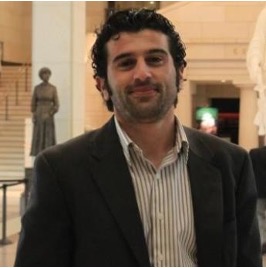
Dr. Falah Al Amro
Advisor: Dr. Hemant Purohit
Institution: George Mason University
Bio: Dr. Amro is an Intelligence Community Postdoctoral Fellow at George Mason University under the mentorship of Professor Hemant Purohit in the Information Sciences & Technology Department. His current research interest centers on leveraging computational and social sciences to study human identity and behavior, specifically using Eigenbehaviors and ensemble machine learning techniques to identify psychological profiles of individuals and groups using multimodal data (i.e. location information, audio cues, body language information, textual correspondence, etc.). Previously he worked as a linguist, instructional technology designer, and a field support engineer. He earned his PhD from George Mason University where he majored in Learning Technologies Design Research.
Abstract: Understanding human behavior and the drivers behind it are of key importance in numerous fields. Unfortunately, human behavior is more complex than simple decision making models imply. Behavior is influenced by based personality traits, historical influences and experiences, situational dependent information and external influences, and a small amount of randomness. In the past research has focused on decision mapping, modeling on single streams of information, and eigenbehaviors. These approaches fail to account for contextual information and fail to fuse together multiple available data streams. In order to appropriately map and understand an individual’s unique psychological makeup we seek methods that broaden the focus using multimodal data (i.e. location information, audio cues, body language information, textual correspondence, etc.) to provide a psychological correlation of the individual. It is incumbent upon the Intelligence Community (IC) to understand human behaviors at their deepest levels because these behaviors drive every aspect of human interaction. While the IC is interested in country scale behavioral dynamics, we also seek to better understand the subject level behaviors that inform and drive those higher-level dynamics. This research seeks to resolve that gap in two ways. First, it will explore methodologies for benefiting from the additional information other modalities provide. Second, it will explore generalization of these methodologies to enable rapid incorporation of new data sources in the future. This work will take a step toward resolving/informing future research and development.