Meet Dr. Rachel Davidson
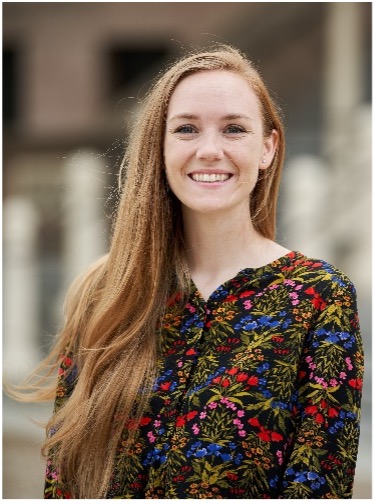
Dr. Rachel Davidson
Advisor: Dr. Sarbajit Banerjee
Institution: Texas A&M University
Bio: Rachel Davidson is currently an Intelligence Community Postdoctoral Fellow in the Department of Chemistry and the Department of Materials Science and Engineering at Texas A&M University working under the advisement of Prof. Sarbajit Banerjee. Her work has focused on understanding electrodeposition of light metals to gain insight on dendrite formation and design of metal anodes for magnesium-based batteries as well as on design of nanocomposite coatings for corrosion protection of light metal alloys. As an IC postdoctoral fellow, she is working to design frameworks which can accelerate the exploration of synthetic design spaces by coupling design of experiment techniques and machine learning in an iterative approach using active learning techniques. She completed undergraduate studies at the University of Alabama at Birmingham. She is a recipient of The Mineral, Metals & Materials Society (TMS) Light Metals Division Best Poster Award, Sigma Xi Grants in Aid of Research Award, Data-enabled Design and Discovery of Energy Materials National Science Foundation Research Traineeship (NRT) fellowship, and was a 2020 Cottrell Scholars Fellow.
Abstract: Machine learning has been strongly embraced by materials scientists looking to systematically explore processing—structure—property relationships in order to accelerate the discovery of new materials. A significant gap in data-driven approaches in materials science is the absence of an adequate number of descriptors and knowledge to establish meaningful and predictive process—structure—property (PSP) relationships. While standard, general-purpose machine learning has been adapted with some modest measures of success, the solution to the underlying problems associated with the discovery and exploitation of structure-processing-function relationships requires the development of de novo data science frameworks that can meaningfully glean understanding from sparse data and their coupling to accelerated materials synthesis and characterization platforms to accelerate the process of discovery and technology innovation. Our approach involves leveraging design of experiment techniques and automated synthesis and analysis platforms to generate strategically designed initial datasets which can be modeled using machine learning algorithms. These initial models are then utilized by active learning strategies to direct future sampling towards underexplored areas which may contain undiscovered materials, improve understanding of the underlying process-structure-property relations at play, or produce materials with the most ideal set of properties. We aim to explore two spaces which present different challenges and constraints in design of synthesis workflows unique to the materials synthesis community. Through the exploration of metastable transition metal oxide synthesis spaces and the systematic design of corrosion inhibiting nanocomposite coatings, we will work to generate an atlas for deterministic mapping of process-structure-property relations.