Meet Alnur Ali
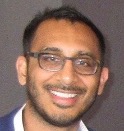
Dr. Alnur Ali
Advisor: John C. Duchi
Institution: Stanford University
Bio: Alnur is a postdoctoral scholar at Stanford University, where he is affiliated with the departments of electrical engineering and statistics. At Stanford, Alnur works with John Duchi, Stephen Boyd, and GuentherWalther on a variety of topics—theoretical, algorithmic, and applied, in nature—at the interface of statistics and optimization. He enjoys thinking about the various problems that arise when trying to get machine learning to “really work” in practice. He received his Ph.D. in machine learning at Carnegie Mellon University, where he worked with Ryan Tibshirani, on algorithmic regularization and sparse regression. Before graduate school, Alnur worked for several years at Microsoft and Microsoft Research, focusing largely on applying machine learning to improve Microsoft’s Bing search engine.
Abstract: Historically, the machine learning community has focused rather intensely on essentially one singular goal: improving sheer predictive accuracy. However, as we increasingly deploy statistical models into mission-critical applications, there is growing recognition that the challenges related to the ongoing monitoring and maintenance of deployed models are equally important. In recent work, my collaborators and I present inferential methodology for the localization and detection of interpretable -- yet anomalous -- subpopulations (i.e., "slices"), where deployed model performance has begun to degrade. Naturally, these slices are useful for continuously monitoring model performance, retraining to boost performance, as well as detecting anomalies. Theoretically, we prove several results showing that our methodology is optimal for recovering latent subpopulations, and for refitting models to improve accuracy (in a structured normal means setup). We present empirical results with three real-world data sets, including a time series involving forecasting the incidence of COVID-19, supporting these claims, and emphasizing the importance of slice-driven model monitoring and maintenance.