Meet Robert Michael Weylandt
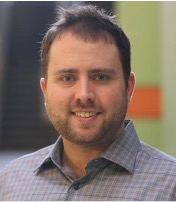
Dr. Robert Michael Weylandt
Advisor: George Michailidis
Institution: University of Florida
Bio: Michael Weylandt is currently an Intelligence Community Postdoctoral Fellow, working with George Michailidis at the University of Florida. His work focuses on statistical machine learning theory for structured multivariate time series, viewed through a graphical model lens. His work has been recognized with best paper awards from the American Statistical Association in both Statistical Learning and Data Science and in Business & Economic Statistics. He has served as a mentor in the Google Summer of Code program for 7 years on behalf of the R Foundation for Statistical Computing and previously held an NSF Graduate Research Fellowship. Prior to beginning his Ph.D. studies, he worked at Morgan Stanley as a quantitative analyst, focusing on derivatives pricing and financial risk management. He received a Bachelor's of Science in Engineering from Princeton University in 2008 and a Ph.D. in Statistics from Rice University in 2020.
Abstract: Graphical or network models help us to understand large-scale networks in a wide range of domains such as communications, social media, sensors, national security, finance, systems biology, and the brain, among many others. These networks can be directly observed, such as a physical sensor network or a digital social media network, or statistically inferred, such as genetic regulation or brain region activation networks. For many of these application areas, we have more information than a single network; for example in social networks, this could be additional demographic or other information on users or multiple networks based on different social media. My research focuses on integrating information from multiple network data sources to find patterns and commonalities as well as irregularities and aberrations. My approach organizes network information as a tensor or higher-order array and develops new analytical tools with theoretical guarantees for extracting meaning from this large-scale network data.
My secondary research interests include machine learning fairness, convex optimization theory, and statistical finance.