Meet Laurel Orr
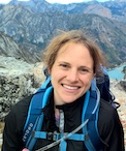
Dr. Laurel Orr
Advisor: Christopher Ré
Institution: Stanford University
Bio: I am a PostDoc at Stanford working with Chris Ré. In August of 2019, I graduated with a PhD from Paul G Allen School for Computer Science and Engineering at the University of Washington as part of the Database Group advised by Dan Suciu or Magdalena Balazinska.
My research interests are broadly at the intersection of machine learning and data management. I focus on how to manage the end-to-end lifecycle of self-supervised embedding pipelines. This includes problems of how to better train, maintain, monitor, and patch the embedding models and their use downstream.
My recent work has centered on building a system for Named Entity Disambiguation called Bootleg that extracts entities in text and generates entity embeddings for use downstream. The insight behind Bootleg is to leverage structured data in the form of entity types and relationships to reason over rare entities that are infrequent in training data but common in deployed settings.
I am a 2020 winner of the IC Postdoc Research Fellowship Program and am one of the 2015 winners of the NSF GRFP in Computer Science. In the summer of 2016 and 2017, I interned at Microsoft Research as a PhD research intern, and in the summer of 2015, I interned at Tableau as a software developer. From the summer of 2012 to the spring of 2015, I interned at Sandia National Laboratories working on high performance computing and image reconstruction.
My goal is to go into academia as a professor of Computer Science after my Postdoc.
Abstract: Laurel Orr is broadly interested in entity-centric AI, from extracting entities to using them in downstream machine learning pipelines. Her current work focuses on addressing two problems in entity-centric pipelines: resource poor domains and handing updates to entity data. In resource poor domains, there is a lack of data available to train entity models and use them downstream. Her work tries to understand how to leverage available metadata and pretrained models to improve performance. Secondly, entity data is constantly changing with new facts being added every day. Entity models need to be updated quickly without sacrificing performance. Her work develops alternative methods to update models such that subsets of entities can be updated at one time while preserving relationships between all entities.