Meet a Participant: Dipnil Chakraborty
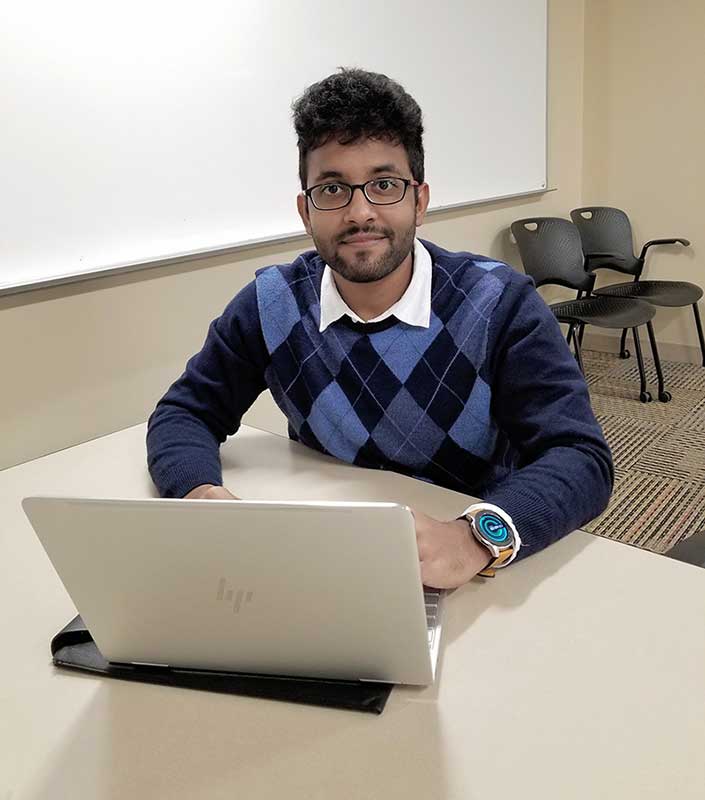
Dipnil Chakraborty, a doctoral student of statistics and two-time participant in the National Science Foundation’s (NSF) Mathematical Sciences Graduate Internship (MSGI) Program, conducted research in predicting traffic flow. Chakraborty’s research contributes to the understanding of accurately analyzing traffic data and improving navigational technologies.
Improving the commute: doctoral statistics student helps advance traffic data analysis
Dipnil Chakraborty is entranced by statistics. Drawn to the practical applicability of the topic, Chakraborty pursued statistics while earning his bachelor’s and master’s degrees in his home country of India and while pursuing a doctoral degree in the United States. “I am spellbound by the charms this subject has to offer,” said Chakraborty.
Chakraborty, a doctoral student at the University of Texas at Dallas, has been a two-time participant to the National Science Foundation’s (NSF) Mathematical Sciences Graduate Internship (MSGI) Program.
The NSF MSGI program offers research opportunities for mathematical sciences doctoral students to participate in internships at national laboratories, industries and other facilities. NSF MSGI seeks to provide hands-on experience for the use of mathematics in a nonacademic setting.
During his second internship with the program, Chakraborty traveled to Argonne National Laboratory in Lemont, Illinois, to perform research under the mentorship of Prasanna Balaprakash, Ph.D., in the Mathematics and Computer Science Division.
Chakraborty investigated methods used to predict traffic flow. Research in predicting traffic and monitoring real-time traffic has become a large area of study in the past several years. Widespread use of smartphones has made navigational apps more popular than ever, and their users want the most accurate and reliable information for their commutes.
Because predicting traffic patterns relies on time-dependent data, researchers typically use recurrent neural networks (RNNs) to analyze data and make predictions. RNNs are a form of machine learning capable of analyzing temporally dynamic data in a sequence, like traffic flow. However, recent research in the field has shown that temporal convolution networks (TCNs), another form of machine learning, may outperform RNNs in processing sequential time-dependent data. TCNs are generally faster and easier to utilize, making them a desirable option among researchers. Chakraborty’s goal was to compare the two methods and determine which performed the best.
Chakraborty spent each day at the lab developing his experimental code of the neural networks, which he then ran on supercomputers. Before his internship, Chakraborty was unfamiliar with analyzing traffic data and the complicated research that is involved in its prediction. Chakraborty not only gained skills and knowledge in the field, but his research demonstrated that TCNs actually can outperform RNNs in analyzing temporal data.
Recalling his internship experience, Chakraborty said, “The computing facilities and the brains that surround you and that you get to collaborate with are an experience of a lifetime.”
Chakraborty returned to the University of Texas at Dallas to pursue his doctoral degree. He credits his doctoral adviser, Sam Efromovich, Ph.D., for his success in pursuing such a challenging and rewarding internship experience such as NSF MSGI. He highly recommends the program to others looking for real-world experience in mathematics. “This program gives you the opportunity to research in laboratories equipped with the best facilities imaginable. For those who are eager for knowledge and research, it is one of the best programs to apply to,” Chakraborty said.
The NSF MSGI Program is funded by NSF and administered through the U.S. Department of Energy’s (DOE) Oak Ridge Institute for Science and Education (ORISE). ORISE is managed for DOE by ORAU.