Meet a Participant: Hrishabh Khakurel
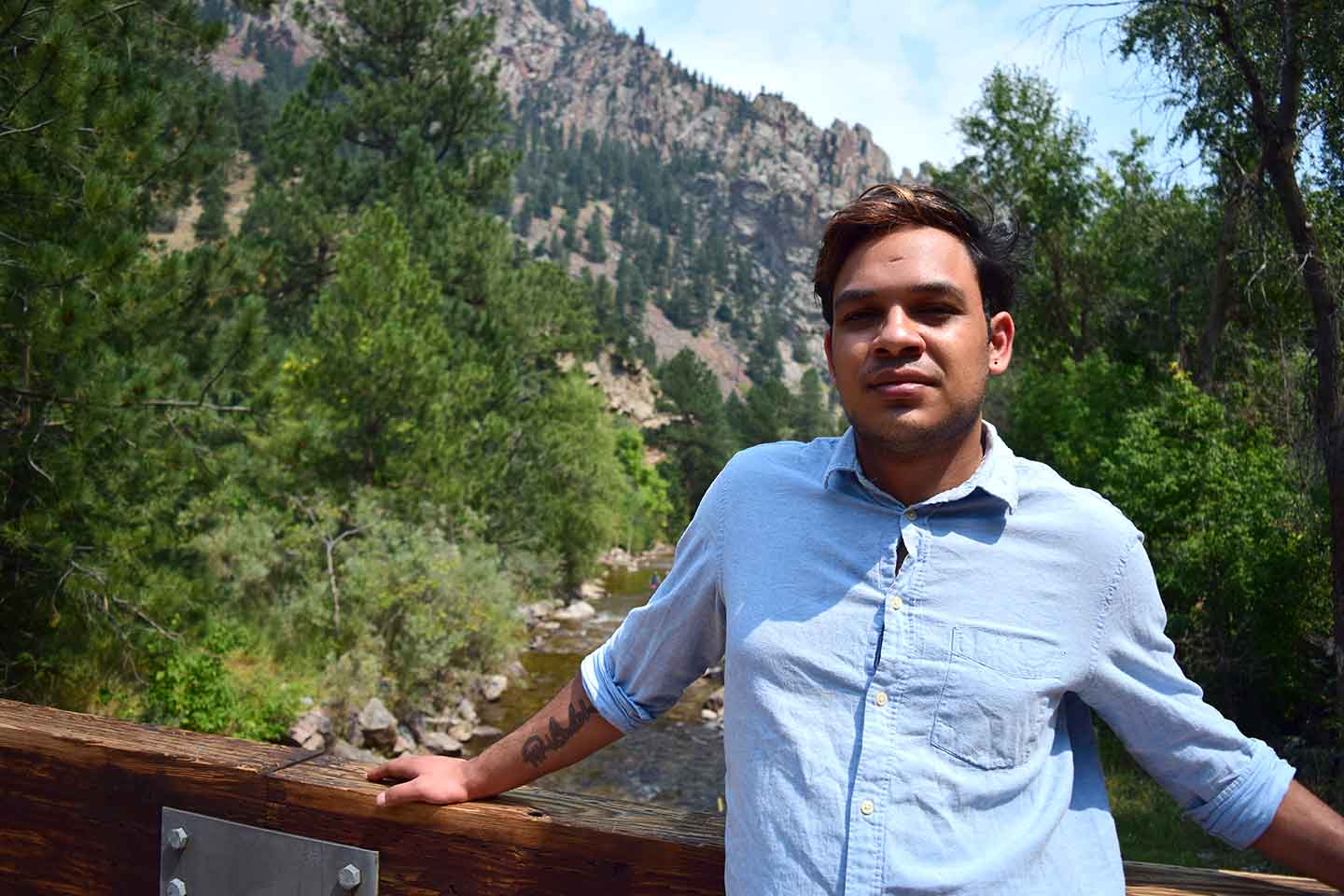
Hrishabh Khakurel, doctoral candidate in statistics and recent participant in the National Science Foundation Mathematical Sciences Graduate Internship Research Participation Program, spent the summer of 2020 analyzing the strength of compositionally complex alloys using machine learning algorithms. (Credit: Ameet Subedi, The University of Texas at Arlington)
Molybdenum Man: Statistician applies machine learning to develop compositionally complex alloys with super strength
Growing up in his hometown of Kathmandu, Nepal, Hrishabh Khakurel cultivated a desire to pursue a field of research that would directly enhance people’s lives. “I chose to study science, technology, engineering and mathematics (STEM) and was attracted to statistics because it is universally applicable in almost every field – from finance to healthcare to governance,” he said.
As a current doctoral student in statistics at the University of Texas at Arlington and former participant in the National Science Foundation’s (NSF) Mathematical Sciences Graduate Internship (MSGI) Research Participation Program, Khakurel spent 10 weeks in the summer of 2020 contributing research to predicting the properties of compositionally complex alloys (CCAs) using machine learning algorithms.
“Alloys are used everywhere from nuclear reactors to car engines,” he said. “Technological advances are based on research to determine which alloys have desirable qualities such as high-temperature strength, corrosion resistance and hardness, making this an exciting research initiative.”
The NSF MSGI Program provides research opportunities for mathematical sciences doctoral students, allowing them to participate in internships at national laboratories, industries and other facilities. NSF MSGI seeks to provide hands-on experience for the use of mathematics in a nonacademic setting.
Under the mentorship of Dr. Ram Devanathan, computational materials scientist and group leader in the Energy and Environment Directorate at Pacific Northwest National Laboratory (PNNL), Khakurel participated in research alongside a team of scientists from three universities and two other national laboratories. This team, led by Ames Laboratory and funded by the U.S. Department of Energy’s (DOE) Advanced Manufacturing Office, focused on accelerating the development of alloys that have superior mechanical performance and can resist oxidation during service at temperatures in excess of 1,200° C due to a novel glassy coating technology.
“Alloys are materials created by mixing multiple metallic elements such as Molybdenum (Mo), Chromium (Cr), and Vanadium (V),” Khakurel explained. “CCAs are made of more than two principal metallic elements and depending upon the use of these alloys, they can have improved properties like strength at high temperatures, corrosion resistance, hardness, etc. compared to conventional alloys.”
Currently, most of these properties are either determined by experimentally preparing the alloys and then measuring these characteristics or they are calculated using theoretical concepts. Khakurel’s NSF MSGI research project, titled Machine Learning Assisted Young’s Modulus Prediction of Compositionally Complex Alloys, focused on using machine learning algorithms to predict the modulus of elasticity, or Young’s modulus, of CCAs.
“The research group I participated with at PNNL incorporated machine learning techniques to predict these properties using datasets drawn from experiment and theory,” Khakurel said. “During my NSF MSGI research project, I used various machine learning algorithms, such as Gradient Boosting, Ada Boost, XGBoost, Random Forest, LASSO and Ridge Regression, to predict the elasticity of these alloys. I compared the accuracy of these models and figured out what kind of training data would give the highest accuracy.”
Compositional optimization is a challenging task to obtain the desired properties of CCAs. Therefore, machine learning is a promising tool to accelerate the search and design of new materials due to its rapidity, scalability and reasonably accurate material property predictions.
“The results of our implementing machine learning tools to predict the elastic modulus of compositionally complex refractory alloys suggest that the difference in atomic radius and valence electron concentration are the key physical features dominating the strength of CCAs and refractory alloys,” Khakurel said. “Using physical laws and domain knowledge made the results easy to interpret. We believe that our machine learning model will open a new avenue to explore the desired material properties for different engineering applications.”
Khakurel had the opportunity during his appointment with NSF MSGI to gain insights from PNNL’s materials scientists about their field, enhanced his skills in machine learning and also attended professional development events such as separate presentations about effective LinkedIn account creation and management as well as on active listening. His research has resulted in two publications that are being submitted to high-impact scientific journals.
Upon completing his doctoral program in statistics, he plans to work in fields related to data science and machine learning.
Khakurel recommended the NSF MSGI program and encouraged others who may be interested, saying, “This is an extremely valuable experience and I recommend that anyone who has the chance to participate in this program not to miss it.”
The NSF MSGI Program is funded by NSF and administered through the U.S. Department of Energy’s (DOE) Oak Ridge Institute for Science and Education (ORISE). ORISE is managed for DOE by ORAU.