Meet a Participant: Justine N’Gozan
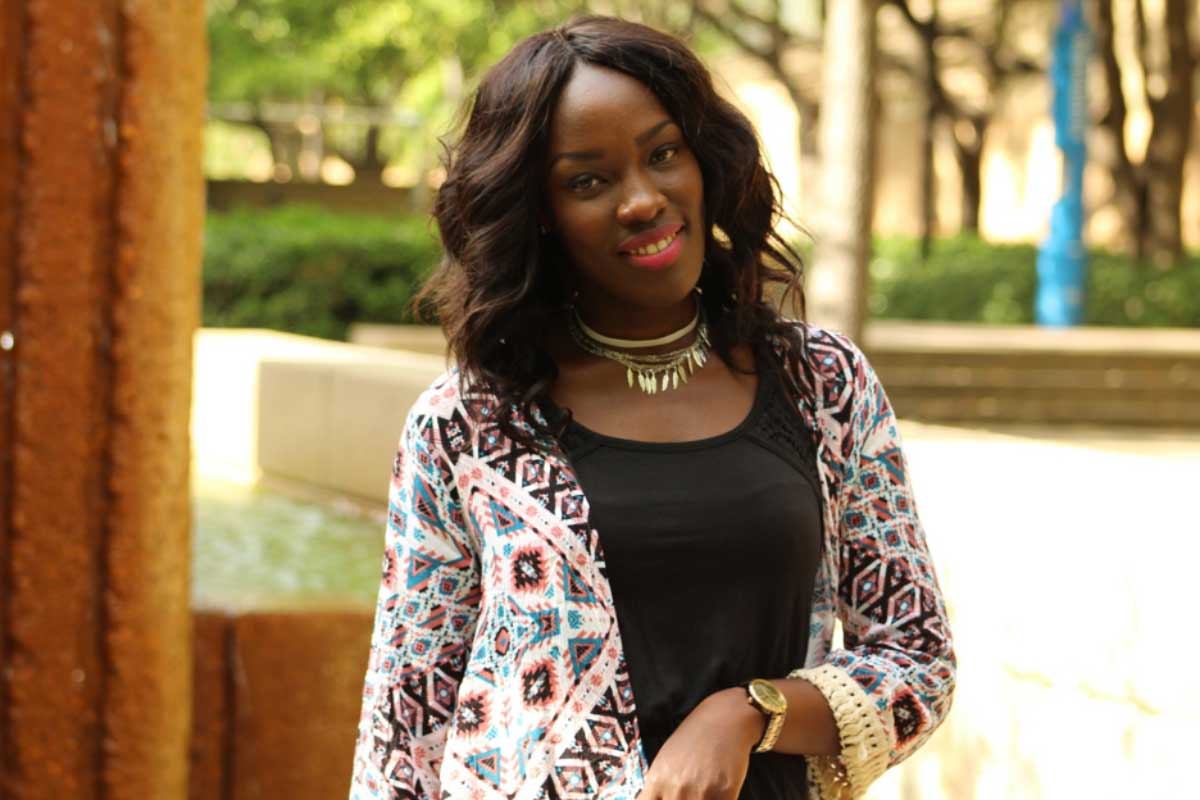
Doctoral student’s research could improve trust in machine learning
Every year, thousands of individuals are diagnosed with chronic diseases like obesity, diabetes and hypertension. While medical practitioners and pharmacists work on creating new, cost-effective therapies to treat these diseases, engineers, data scientists and other researchers like Justine N’Gozan are studying deep learning algorithms that could help identify and understand at-risk patient populations.
“Increasingly, machine learning algorithms are crunching huge amounts of data and identifying patterns that humans cannot,” said N’Gozan, a first-year mathematics doctoral student at the University of Texas at Arlington.
“Unfortunately, there is a fair amount of distrust in applying predictive models to domains such as medicine, criminal justice and finance, where humans are directly impacted. Therefore, it is critical to understand the reasoning behind decisions made by deep learning systems so we can translate decisions into actions,” she said.
N’Gozan served as a recent intern in the National Science Foundation’s (NSF) Mathematical Sciences Graduate Internship (MSGI) Program at Lawrence Livermore National Laboratory in Livermore, California. She was tasked with developing new statistical learning algorithms to interpret complex machine learning models.
The NSF MSGI program provides research opportunities for mathematical sciences doctoral students to participate in internships at national laboratories, industries and other facilities. NSF MSGI seeks to provide hands-on experience for the use of mathematics in a nonacademic setting.
Under the mentorship of computer scientist Jayaraman J. Thiagarajan, Ph.D., N’Gozan used the Python programming language to develop algorithms to dissect and understand the functioning of a convolutional neural network. These networks are used for image classification applications, such as facial recognition software on smartphones.
N’Gozan spent her time writing and implementing code. By the end of the internship, she successfully developed a method that could be useful in predictive analysis applications.
“The results from this study provide novel statistical tools that will enable analysts to obtain a holistic view of predictive models and potentially help build trust in both individual predictions and models overall,” said N’Gozan. She and Thiagarajan plan to present the research at an upcoming machine learning conference.
The NSF MSGI program granted N’Gozan the unique opportunity to blend classroom knowledge with real-world application, an experience that boosted her confidence and honed her research and communication skills. In addition to conducting research, she discussed research goals with her mentor, and she attended workshops and lectures pertinent to machine learning.
“One of my lifelong goals is to engage in ongoing learning and improvement. I hoped this program would allow me to enrich my self-exploration and reflect on my intellectual journey, and it did,” N’Gozan said.
“I evaluated my goals and acquired new knowledge and skills that will be useful in my future endeavors. I am now more excited than ever to do research and explore new topics. I will definitely recommend this experience to others,” she said.
The NSF MSGI Program is funded by NSF and administered through the U.S. Department of Energy’s (DOE) Oak Ridge Institute for Science and Education (ORISE). ORISE is managed for DOE by ORAU.