Meet a Participant: Hang Deng
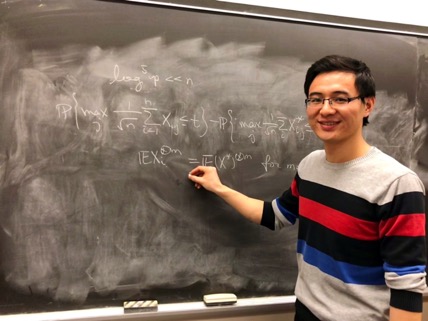
Using Statistics and Deep Learning to Improve Women’s Health
Cervical cancer is the fourth most common cancer in women globally, with an estimated 528,000 new cases and 265,700 deaths per year according to the World Health Organization. In the United States, the cervical cancer death rate has decreased by more than 50 percent in the last 40 years with the use of the Papanicolaou (Pap) test, one of the most effective ways to detect pre-cervical cancer. However, more than 4,000 women each year die from cervical cancer in the United States. Globally nearly 85 percent of cases of cervical cancer occur in less developed regions, where access to screenings is not common.
The best way to prevent cervical cancer is through regular screenings, but not every country has the same resources for screenings or the same process of administering them. Using Norway as a case study, doctoral student Hang Deng applied his statistical knowledge to better understand the process of cervical cancer screenings and possible ways to improve testing frequency. His research was made possible by the National Science Foundation’s (NSF) Mathematical Sciences Graduate Internship (MSGI) Program.
The program provides research opportunities for mathematical sciences doctoral students to participate in internships at national laboratories, industries and other facilities. NSF MSGI seeks to provide hands-on experience for the use of mathematics in a nonacademic setting.
Deng was stationed at Lawrence Livermore National Laboratory, California, under the mentorship of Ghaleb Abdulla, Ph.D. Deng and Abdulla’s team of researchers analyzed survey data of Norwegian women’s health information with the goal to develop personalized cervical screening policies.
Typically, women between the ages of 20 and 65 receive the recommendation to have a Pap smear test every one to three years. However, by using each patient’s history, a determination may potentially be made to reduce the frequency of testing without losing the ability to successfully detect cervical cancer at the proper time. Safely reducing the frequency of testing would cut medical screening costs and lessen the interference of testing in women’s lives.
To achieve their goal, Deng and the team adopted a deep learning approach using a model called long short-term memory (LSTM) neural networks. Deep learning is a new area of machine learning methods. LSTM uses a technique that allows learning to occur over many time steps, training the system using a large amount of data.
Deng used a significant amount of women’s health data, as well as knowledge from previous trainings on LSTM neural networks to help train the current model specifically for cervical cancer. By inputting data including women’s previous screenings and test results, LSTM could begin to learn and predict personalized screening recommendations. The methodology developed by the team is applicable beyond the Norwegian women in the study. The methodology can also be applied to similar data in other countries for cervical cancer or other diseases that share the same medical data structure.
“The project has a truly useful application,” Deng commented. “I was highly motivated when I realized that we may actually make a difference in Norwegian women’s lives and hopefully more people’s lives later on.” During his internship, Deng had the opportunity to explore deep learning methods and discover new areas of research.
“The experience was great and unforgettable because it helped me broaden my research horizons. I was able to meet many people with different backgrounds and areas of expertise. I learned a lot from discussions with other people,” Deng said.
Deng returned to Rutgers University where he plans to graduate with his doctoral degree in 2020.
The NSF MSGI Program is funded by NSF and administered through the U.S. Department of Energy’s (DOE) Oak Ridge Institute for Science and Education (ORISE). ORISE is managed for DOE by ORAU.