Meet a Participant: Yinpu Li
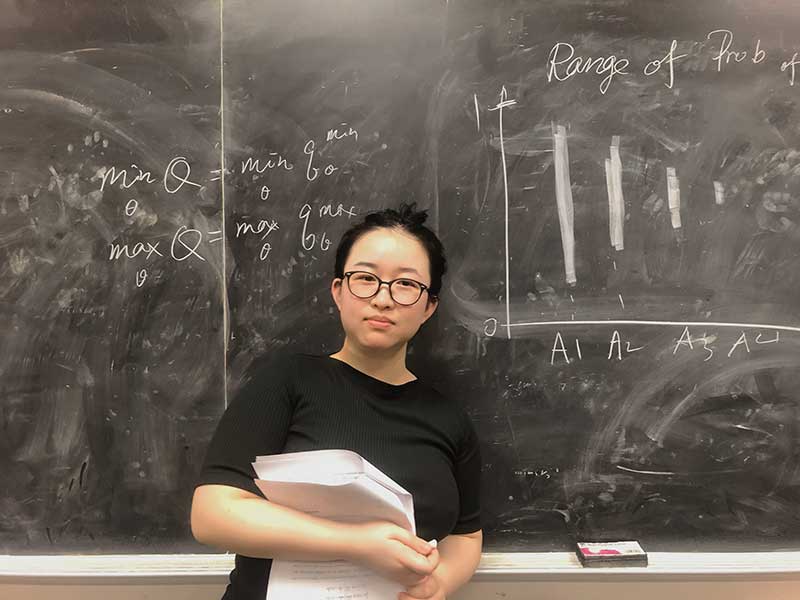
Yinpu Li, doctoral student in statistics, spent the summer contributing to uncertainty assessment research within the National Science Foundation’s Mathematical Sciences Graduate Internship Program. (Credit: Yinpu Li)
Statistician spends summer contributing research to statistical uncertainty assessment
Growing up, Yinpu Li gained a lot of confidence in mathematics and found statistics to be a good tool to explore the world and satisfy her curiosity in revealing the ground truth in nature. While earning her doctoral degree in statistics at Florida State University, and as a recent participant in the National Science Foundation’s (NSF) Mathematical Sciences Graduate Internship Program (MSGI), she seized upon the opportunity to apply that confidence and curiosity while contributing to research involving uncertainty quantification and statistical probability modeling.
The NSF MSGI Program provides research opportunities for mathematical sciences doctoral students, allowing them to participate in internships at national laboratories, industries and other facilities. NSF MSGI seeks to provide hands-on experience for the use of mathematics in a nonacademic setting.
When Li heard about the NSF MSGI Program while earning her master’s in statistics at Florida State University, she became interested in how the program embraced frontier scientists in a national lab setting and thought it would be a great opportunity to learn from them through mutual collaboration.
Once she was accepted into the program, and under the mentorship of Hari Iyer and Steve Lund, mathematical statisticians at the National Institute of Standards and Technology (NIST), Li contributed statistical research on probability and decision theory.
“The theory of statistics helps us in developing reasonable statistical models to explain observed phenomena,” she said. “At the same time, the theory of probability provides methods for a systematic analysis and assessment of probabilities for events of interest while decision theory helps us in making optimal decisions under uncertainty.”
Using the theory of probability and statistics, Li focused her research on constructing a framework to better understand uncertainty when making decisions in light of unavailable data regarding events of interest.
“Unfortunately, there is no single, correct model to describe observed data. Instead, prior knowledge and/or data are quite often used to develop models,” she said.
“Therefore, a proper understanding of our ability to assess the probability of an event can be gained only by exploring how the results change as we explore the space of all possible models,” she said.
Li’s research project at NIST used numerical approaches to address model uncertainty and develop uncertainty pyramids. Model uncertainty is uncertainty due to approximations and idealizations made when representing real world phenomenon within a mathematical framework, as well as in the choices one makes when selecting a probability model for the representation of uncertainties.
“A comprehensive model uncertainty assessment tool was developed for the case where inferences are made based on independent and identically distributed univariate data,” she said.
Advancing research concerning uncertainty quantification is important to better understand statistical inference problems such as in forensic science and criminal cases.
“The standard approach to uncertainty quantification usually underestimates the actual uncertainty,” Li said. “There are many real life problems where it is critical that the level of uncertainty associated with inferences is as fully characterized as possible, particularly when assessing the reliability of systems whose failure can result in loss of life, property or individual freedom.”
While Li’s research project is ongoing and will require more real data to test the methods she helped to develop, she was encouraged by her experience and motivated to remain persistent in her future research. “We were able to implement our ideas in simple, univariate situations under the assumption of independent and identically distributed data, yet much remains to be done for more complicated cases,” she said.
During her internship, Li learned comprehensive and systematic model uncertainty assessment concepts to integrate into her doctoral dissertation and was excited to collaborate with first-class researchers in non-academic institutions.
“My interactions with colleagues having different backgrounds and various skill-sets motivated me to be a persistent and critical researcher,” she said.
Her internship also imparted new concepts and skills such as more comprehensive and systematic tools to investigate model assumptions, further critical views of uncertainty quantification in statistical reasoning and better optimization methods to go along with those views.
“The experience at NIST was a great encouragement to me,” Li said. “By participating in the NSF MSGI program, I’ve gained the first-hand experience of mathematical computation and statistical reasoning in a non-academic setting while learning new concepts from forensic science.”
Li also took the opportunity during her appointment to attend seminars hosted by NIST in the Statistical Engineering department and seized upon the chance to present her research on Bayesian additive regression trees models for nonparametric problems.
Li recommended the program for others, saying, “I really appreciated this opportunity and have benefitted a lot from the experience.”
“My mentors not only mentored me and helped me improve upon the project, but also inspired me by sharing their experience about their own career which is a good example when my career is at such an early stage,” she said.
“Additionally, the critical views from these frontier researchers and scientists boosted my interest in working in a non-academia research institution,” Li said.
The NSF MSGI Program is funded by NSF and administered through the U.S. Department of Energy’s (DOE) Oak Ridge Institute for Science and Education (ORISE). ORISE is managed for DOE by ORAU.