Meet a Participant: Wisdom Ogala
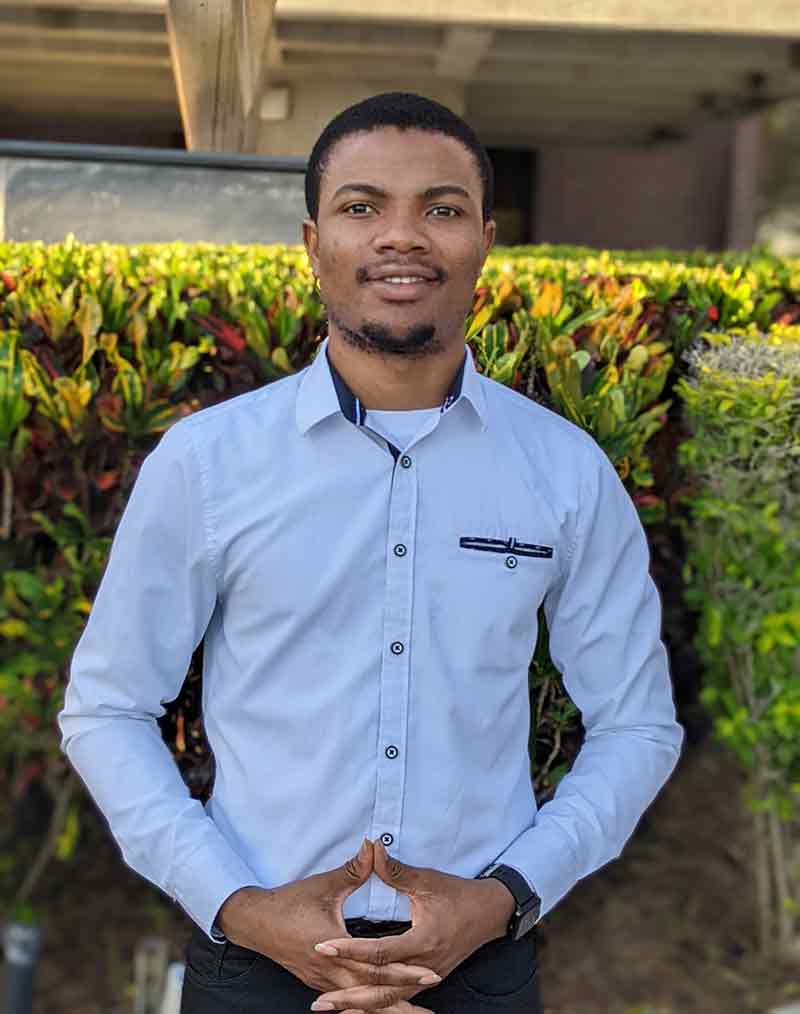
Wisdom Ogala, doctorate student in mathematics, contributed to the application of machine learning and the development of change point detections for forecasted power-grid frequencies to help prevent power downtime. (Photo Credit: Comfort Omonkhodion, University of Central Florida)
Machine learning predicts future power grid outages to help save lives
Math is in everything from art, to economics and even the energy grid, and Wisdom Ogala is certain it can be used to more efficiently predict power outages.
Ogala was first interested in the applications of math after his high school teacher told him that math was used by great thinkers. This inspired him to go to college and learn more about its real world usage. He studied for his masters in mathematics in Europe and is currently in his third year at the University of Central Florida studying for his Ph.D. in the financial math track.
He saw how the National Science Foundation’s (NSF) Mathematical Sciences Graduate Internship (MSGI) Program aligned with his values and curiosity, so applied to be an NSF fellow. The NSF MSGI Program provides research opportunities for mathematical sciences doctoral students, allowing them to participate in internships at national laboratories across the country. NSF MSGI seeks to provide hands-on experience for the use of mathematics in a nonacademic setting.
Ogala spent ten weeks putting his knowledge to the test. He explored how machine learning could be used to improve the predictive capability of the energy industry when it came to power outages.
“Power outages cripple economic activities,” said Ogala. “The 2021 Texas power outage, caused by severe winter storms, lasted for 17 days, claimed hundreds of lives and cost no less than 196 billion dollars in property loss.”
Along with his mentor, Amanda Lenzi, Ogala developed a deep learning architecture that can read previous power-grid frequency data, predict future power-grid frequencies, and detect abrupt changes in the predicted power-grid frequencies. Deep learning is an artificial intelligence algorithm that can collect data and form predictions. This helps the operators respond and isolate problems before they snowball. For instance, they might turn generators on to increase output, or reduce energy consumption when there is a predicted decline in system frequencies.
They accomplished this by observing data, writing code, and adjusting the performance of the deep learning algorithms. With a convolutional neural network (CNN) combined with Long-Short Term Memory (LSTM) studying data patterns they were able to decide on a normal power frequency baseline and find frequencies that fall outside of the normal range. However, Ogala noted that this was not very successful at first. Because the data ranges were so small it was difficult for the machine algorithms to learn and find the pattern of predictability. To solve this problem, data standardization was more than necessary.
Ogala explained how they adapted to this issue. “We learned how to manage the low variational data problem by employing various standardization measures, development of sophisticated CNN-LSTM and using Neural Network Intelligence Bayesian hyper parameter tuner to choose the best hyper parameters for our model in each search space.”
By the end of his internship, he and his team were able to successfully predict outages up to ten seconds in advance, which Ogala believes could be further improved over time.
Ogala presented his findings at the annual Argonne National Laboratory Summer Argonne Students Symposium event.
During his internship Ogala was glad to gain experience in a new field, learn persistence and enjoyed going to weekly seminars that expanded his scope of thinking. His favorite part of the experience was being welcomed in by the lab and the weekly get-togethers he attended.
Looking forward beyond graduation, Ogala plans to go into quantitative research and intends to apply what he learned about machine learning in his quantitative algorithms.
“Overall, I had an awesome experience in NSF-MSGI,” Ogala finished.
Math is in everything, and thanks to the research by great thinkers like Ogala, it is hopeful that math will one day help the nation better react to and mitigate power outages.
The NSF MSGI Program is funded by NSF and administered through the U.S. Department of Energy’s (DOE) Oak Ridge Institute for Science and Education (ORISE). ORISE is managed for DOE by ORAU.