Meet a Participant: Olga Khaliukova
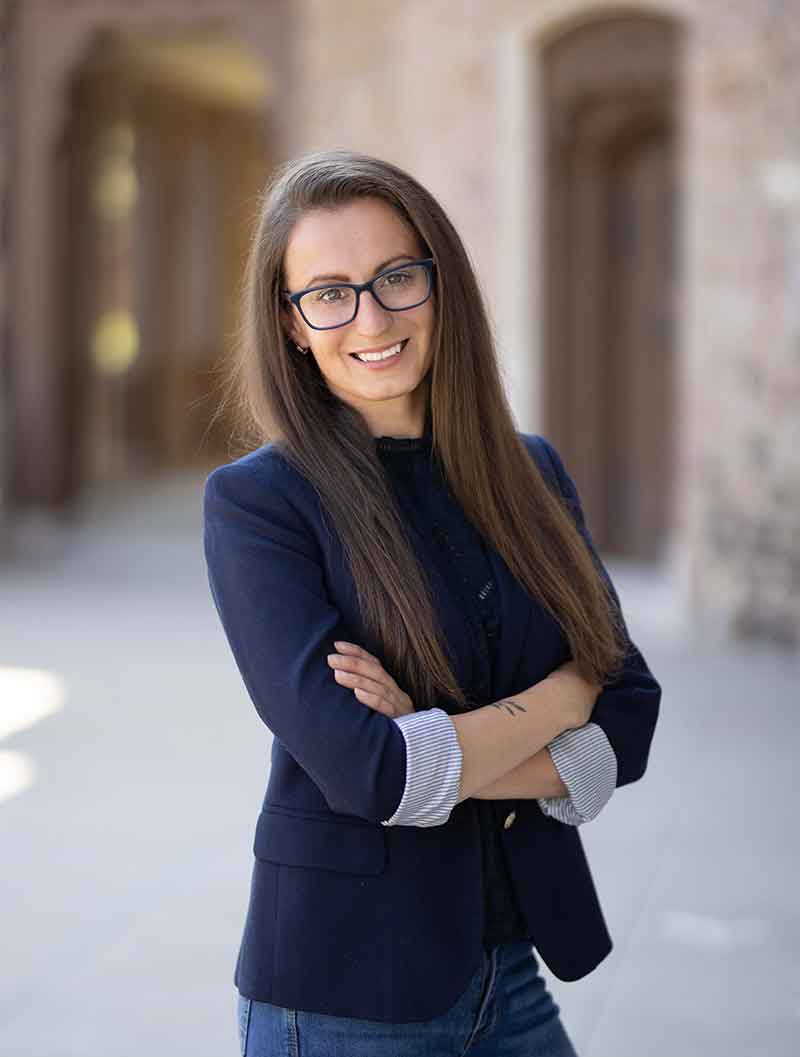
Olga Khaliukova researches how machine learning algorithms, such as deep neural networks, can be used to improve microscopy imaging techniques. Her research can help laboratories provide more accurate microscopy imaging test results. (Photo Credit: Angela Prodanova, Proda Photography)
Statistician uses machine learning to improve microscopy imaging techniques
“Sometimes talking to one person who believes in you and your capabilities is all that it takes to start dreaming big and taking action,” enthused Olga Khaliukova as she began her story.
Khaliukova grew up in Kyiv, Ukraine, where her mother worked three jobs as a nurse to support Khaliukova and her older brother. Her brother was her first supporter. She learned the value of hard work from him, but also how to be compassionate and dream big.
“Back then, in Ukraine, young people were mostly choosing a profession that could support the family financially, and they were rarely following their dreams,” she explained. “Having my brother who believed in me was inspiring and comforting. That marks the beginning of my passion for mathematics.”
When Khaliukova was eleven, her brother was drafted into the army and killed in the line of duty a year later. She and her family grieved, resulting in her mother leaving for Libya to join the Red Cross. Khaliukova stayed behind and put everything into her studies, which she said helped her to stay focused and keep structure in her life. Believing in herself after such tragedy had not been easy.
When it was finally time to choose a path, she chose agriculture because it was the largest industry in Ukraine and would be secure. She would not come back to mathematics until she became a student at the University of Tennessee to receive her masters in agricultural and resource economics, where her professors noticed her passion for math. Because of their support she took a few statistics and math courses while she was there. She is now in her second year at the Colorado School of Mines for her doctoral degree in statistics.
She first heard about the Oak Ridge Institute for Science and Education’s (ORISE) National Science Foundation Mathematical Sciences Graduate Internship Program (NSF MSGI) from her department head at the Colorado School of Mines.
The NSF MSGI program provides research opportunities for mathematical sciences doctoral students, allowing them to participate in internships at national laboratories, industries and other facilities. NSF MSGI seeks to provide hands-on experience in the use of mathematics in a nonacademic setting.
Wanting to put her statistics knowledge to the test, as well as appreciating the NSF’s commitment to diversity, Khaliukova applied and was accepted. She would spend ten weeks of her summer researching and honing her skills as a statistician.
She was placed on a team with her two mentors, Steven P. Lund, Ph.D., and Hariharan Iyer, Ph.D., to bring her expertise to the “Measurement, Description, and Analysis of Patterns” project at the National Institute of Standards and Technology, (NIST). This project looked at the differences between viewing factors for microscopy imaging, specifically differences between multiple machine learning techniques such as random forests and deep neural networks. This was done to find the best way to adjust the microscope settings for high-quality images.
Microscopy imaging is used by laboratories to visualize organisms and environments on the microscopic scale, for example bacteria and fungi.
“One of the challenges of microscopy analysis is significant measurement deviations. The inconsistencies are influenced by differences in supplies (e.g., microscope slide thickness), equipment measurement variations by manufacturer, brand or production series, human factor (e.g., years of experience), and cell behavior that can be altered by intensity and length of exposure to light,” she said.
These inconsistences are what can cause two different labs, which both are provided the same samples, to come back with two different results. This can lead to inconsistences in tests that rely on microscopy imaging.
Machine learning, which is a branch of artificial intelligence, is frequently used by statisticians to analyze data and can assist in improving microscopy imaging analysis. Neural networks are a set of algorithms that are created to recognize patterns in data. Random forest is a supervised machine learning algorithm, which contains multiple decision trees.
“The random forest algorithm provides a higher level of accuracy in predicting outcomes over a single decision tree algorithm. For example, it would be used to classify whether an email is a spam or not,” she said.
Through studying machine learning, Khaliukova hopes to decrease the inconsistences within microscopy imaging techniques, which will improve the consistency and reliability of the microscopy imaging analysis.
She thinks back to her internship proudly. Khaliukova is glad to deepen her knowledge of supervised machine learning algorithms and their architecture, and said she was able to improve her R programming skills, including with the R package imager. R programming, which is an important statistical computing language, is crucial for data scientists and mathematical statisticians to be proficient in.
She also learned from her two mentors the importance of the art of visualization in research findings and presentation skills, saying that while research could be groundbreaking, it still needs to be presented in an appealing way to engage the audience. This includes using creative presentation aspects, such as color choice and form, which she says she uses daily.
Since returning to school after her summer at NIST, Khaliukova is working hard at her Ph.D. She is a member of the Methane Emissions Monitoring team at the Colorado School of Mines, and her research as a student focuses on environmental monitoring using satellites and data monitoring.
She shared her appreciation for ORISE and the NSF MSGI program. She is pleased that she was able to learn and develop her knowledge during the internship.
“You will have a chance to work on emerging issues in your field of interest, deepen your knowledge, and improve your practical skills,” she said. “I would recommend the ORISE program without reservation. The ORISE application process was clear, the instructions were easy to follow, and the support team was easy to communicate with.”
Khaliukova’s story proves how powerful the care and support from family, peers, and those we look up to can be. Thanks to the encouragement she received, along with her own solid determination, her time with the NSF MSGI program will surely help to improve microscopy imaging analysis.
The NSF MSGI Program is funded by NSF and administered through the U.S. Department of Energy’s (DOE) Oak Ridge Institute for Science and Education (ORISE). ORISE is managed for DOE by ORAU.